Requirement for smart in-process control systems to deliver cell therapy processes fit for the 21st century
Cell Gene Therapy Insights 2016; 2(6), 683-689.
10.18609/cgti.2016.074
The cell therapy field continues to experience significant growth with an increasing number of therapies approaching commercialization and over 535 products currently undergoing phase II and III clinical trials [1]. This focus towards commercialization is leading cell therapy innovators to increasingly look at technologies which can support improved product monitoring, allow more controlled processing and increased consistency during manufacturing. These needs are similar to those experienced in conventional bioprocessing where approaches such as quality by design (QbD) are increasingly being applied to achieve enhanced control of therapeutic products such as monoclonal antibodies. QbD incorporates prior product knowledge with the use of statistically designed experiments, risk analysis and knowledge management. The intent of QbD is to develop sufficient understanding of the product to ensure robustness and consistency in the manufacture process. As the cell therapy field continues to mature it is timely to look at how learnings and technologies from related fields can be used to expedite the development of modern cell therapy manufacturing strategies.
Process analytical technology
A key component of the QbD process is the development and implementation of Process Analytical Technologies (PAT). PAT is a framework for “designing, analyzing, and controlling manufacturing through timely measurements (i.e., during processing) of critical quality and performance attributes of raw and in-process materials and processes, with the goal of ensuring final product quality” [2]. The aim of PAT is to obtain better process control by identifying and managing sources of variability, reducing cost by optimizing the use of raw materials and minimising product cycle times through the use of measurements that are in-line (analyzed in place), on-line (sample removed, analyzed and returned to the process stream) or at-line (sample removed and analyzed close to the process stream) [3]. PAT has been successfully applied for chemical pharmaceutical manufacture for decades and is increasingly used to support biopharmaceutical production [4]. However, there are many challenges to applying methods amenable to PAT to support biomanufacture of cell therapy products. For autologous products there is often an inherent variability in the patient specific starting material. This variability may be increased if the cells are obtained from older patient populations who have undergone a range of first line treatments for their malignancies. For example, CD19 chimeric antigen receptor T-cell immunotherapies used for the treatment Non-Hodgkin’s Lymphoma (NHL). More than 90% of these patients are over 65 years old when diagnosed with NHL [5] and may have received initial treatments including single agent alkylators, nucleoside analogues, combination chemotherapy, or monoclonal antibodies [6]. For allogeneic therapies there is the potential to have more control over the heterogeneity of the cellular starting material through the use of characterized master and working cell banks. However, these products are equally affected by other processing variables such as lot-to-lot inconsistencies of raw materials, the activity of growth factors and cytokines, fluctuation in the availability of nutrients and progressive build-up of metabolic waste products.
Implementation of PAT requires a high level of product understanding including the interlink between Critical Quality Attributes (CQAs) of the product, performance attributes of the process, and key characteristics of the various raw and in-process materials. Establishing this level of product understanding takes time and can be gained and refined during the whole process development lifecycle. It has been proposed that PAT should be implemented over several phases of activity [7,8]. The initial phase begins when the unit operations are being designed and optimized during the early stages of product development and involves the identification of the product CQAs and the assessment of the impact of Critical Process Parameters (CPP) on these CQAs. The next phase involves the identification of suitable technologies that can be used to monitor the CQAs and CPPs within a suitable timeframe to allow real-time decision making. The final phase involves the optimization and validation of the technologies to control the CPP’s and demonstrate improved consistency of manufacture.
The PAT challenge for cell therapies
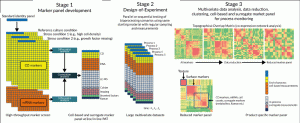
Figure 1. Overview of PAT’s and marker selection procedure. In stage 1, screening of cell-based markers results in smaller product-specific protein and molecular marker panels modulated by changes in culture regimen. This panel is augmented with measurements from suitable in/at-line PAT systems. In stage 2 this panel is used to record changes at multiple time points with measurements correlated to different production processes implemented through a DoE methodology. In stage 3, the resulting multivariate datasets are analysed to identify a set of robust cell-based and surrogate markers. Co-expression network analysis (A) can be used to narrow down a subset of such markers by removing the least significant ones. Alternatively (B), only markers strongly correlated with robust surrogate markers (e.g. “texture” from live cell imaging) can be investigated in order to derive a smaller product-specific panel to be used for in- and end-of-process monitoring.
A fundamental challenge to implementing PAT to support cell therapy manufacture is how to define cell quality. For biopharmaceutical processing the cells are a vehicle to produce a therapeutic agent which can be isolated and purified without the need to recover the cells. As a consequence the implementation of PAT during the culture process is generally straight forward, as the aim is to maintain a viable growing cell population and the window for decision making is relatively wide. By contrast, the challenge for cell therapies is to decipher the relationships between raw material variables (nutrients, growth factors, extracellular matrix substrates), product variables (cell phenotype, metabolic profile, apoptosis etc.) and process variables (medium perfusion or exchange rate, feeding regime, stirring speed, pH, dO2, etc), to ensure that product quality is maximized and CQAs are maintained throughout the process.
This inherent complexity means that current implementation strategies for PAT alone do not provide robust information to address these questions. A gap exists between discrete in-process product characterisation (phenotype, cell stress, cell cycle) and properties usually monitored through PAT which provide a continuum of data but are largely inferential in nature (e.g. dO2, pH, glucose consumption or cell mass). At the Cell and Gene Therapy Catapult, we are bridging this information gap with the introduction of an agnostic multiparametric cell characterization and multivariate data analysis approach.
Our approach is implemented in three stages as outlined below and in Figure 1:
1. Cell-based marker panel development
A standard product identity panel is generated which is composed of 100’s of potential protein and molecular target that are screened using high throughput multiplexed technologies. The screening panels are designed to incorporate both product specific targets and generic markers for cell quality based on stress (e.g. DNA repair, oxidative stress, hypoxia, apoptosis) and metabolic status. A “reference” dataset is generated using material produced following the established process (for which CQAs are known to be maintained) as well as comparator data produced under defined stress-inducing conditions (e.g. high cell density, low abundance of growth factors). Differential analysis between these production runs followed by correlation analysis between the protein and molecular targets allow a smaller panel to be identified which will contain markers reliably modulated by changes in the culture regimen.
2. Design-of-Experiments (DoE)
The cell-based marker panel developed in stage 1 is further augmented with additional measurements from suitable in/at-line PAT systems. These could include technologies such as liquid chromatography mass spectrometry (LC-MS), photometric metabolite analysers, Raman spectroscopy, label-free live cell imaging and protein arrays. These additional PAT’s can typically measure 10’s to 100’s of surrogate markers each. Several production processes are run in parallel or sequentially based on a Design-of-Experiment methodology. Measurements using the full panel from this stage are taken at regular intervals throughout the runs, resulting in large multivariate datasets.
3. Multivariate data analysis [9] (MVDA)
Data modelling techniques such as co-expression network analysis [10] are used to collate the data from the DoE experiment and identify markers whose expression patterns are modulated similarly under the different process conditions. This generates a Topological Overlap Matrix (TOM) which quantifies the correlation and connectivity of each marker with all the other markers. By stringently removing the least connected markers from the TOM dataset a low resolution matrix is produced which contains a refined panel of product specific markers that can be used for in-process monitoring.
This approach is agnostic as there is no theoretical requirement to relate measured changes in marker/signal outputs to the physiological and biochemical nature of the changes. It is therefore amenable to adaptation across a wide range of cell therapy products and processes. The availability of high-throughput quantitative methods using commonly applied platform technologies such as flow cytometers and RT-qPCR is instrumental in establishing robust product fingerprints from which quality attributes can be tested and panels designed for routine analysis.
PAT technology selection
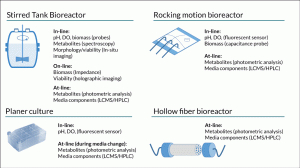
Figure 2. Comparison of the typically available range of in-/on-/at-line PAT measurement technologies that can be applied to culture systems for cell therapy processing.
Selecting suitable analytical technologies to support PAT for cell therapy manufacture is also challenging. Consideration must be given to the capability of the technology to make robust measurements in a complex culture formulation without interference from other components. Ideal technologies should also be capable of supporting real time monitoring or at a minimum provide data in a timeframe sufficient to allow proactive decision making. Sample availability and access to the culture system could also be a limitation. Unlike large scale biopharmaceutical production where the majority of cell culture operations involve the use of stirred tank bioreactors [11], cell therapies are produced using a wide variety of culture systems. These range from open platforms such as multiwall plates, T-flasks, roller bottles, bags and multilayer flasks through to closed bioreactor systems such as hollow fiber, rocking motion, packed bed and stirred tank bioreactors. Some of these systems have probe ports allowing a wide range of technologies to be utilised for bioprocess monitoring while others such as multilayer flasks and hollow fiber bioreactors may limit analysis to the spent media (Figure 2).
The demand for real-time and rapid monitoring within the bioprocessing field has resulted in significant innovation and automation of process analysers [12]. Some of these technologies may be applicable for cell therapy process monitoring (Table 1). However, their implementation is often not straight forward and could require significant capital investment and/or access to specialist expertise. For example, optical sensors such as near infrared and Raman spectroscopy are non-invasive and can provide continual in-line monitoring of parameters associated with cell metabolism such as glucose and glutamine consumption and production of lactate and ammonia. However, analytical data from online optical systems is typically not amenable to direct interpretation. Consequently, chemometric methods using MVDA techniques such as partial least squares are required for effective data analysis [13]. At-line technologies such as mass spectrometry use difference in the mass-to-charge ratio of ionized atoms or molecules to provide detailed information about the concentrations of media component. This information can be provided in near real-time but the systems are expensive to set up and require analytical chemistry expertise to establish the monitoring methods. Other technologies such as fluorescent optical sensors for pH and DO analysis can be cheap to implement and are increasingly being used for in-line monitoring in a range of culture systems. These sensors can provide real time information but measurement reliability can decrease after 15–20 days in culture [14], potentially limiting their use for cell therapies with long manufacture processes.
Table 1: Potential PAT technologies that could be used for cell therapy process monitoring. | ||
---|---|---|
Technology | Measurement | |
In-line | NIR spectroscopy | Glucose/Glutamine/Lactate/Ammonia, VCD/TCD/osmolality |
Raman spectroscopy | Glucose/Glutamine/Lactate/Ammonia, VCD/TCD/osmolality | |
Fluorescent sensors | pH and DO | |
Refractive index | Compositional changes | |
multiwavelength Fluorimetry | Amino acids | |
Holographic imaging | Cell shape/size, cell viability | |
Impedance | Biomass/cell viability | |
Turbidimetry | Biomass | |
In/At-line | HPLC | Media components (amino acids, sugars, proteins, metabolites) |
LC-MS | Media components (amino acids, sugars, proteins, metabolites) | |
Coulter counter | Biomass/cell viability | |
Imaging | Cell size/shape, cell viability | |
Photometric analysers | Glucose/Glutamine/Lactate/Ammonia | |
DO = Dissolved Oxygen; TCD = Total Cell Density; VCD = Viable Cell Density |
Conclusion
As the cell therapy field matures and companies strive to develop cost effective manufacturing strategies, the requirement for better process control by implementing PAT is increasing. This is being underpinned by advancements in analytical screening technologies and the development of multivariate analysis techniques which allow data from several sources to be combined and analysed simultaneously. While the implementation of PAT is not always straight forward and may require access to specialist expertise it does present opportunities to move away from “locked in” processing to more flexible manufacture by monitoring and controlling known sources of variability. As PAT is increasingly applied this will present opportunities for technology miniaturization and the development of single use biosensors to bring more analysis in/at line to further increase consistency of manufacture. Going forward, this ability to produce a dynamic description of the process could allow inherent QC during production, providing opportunities for “right first time” manufacture and potentially supporting real time product release.
Financial & competing interests disclosure
Cell and Gene Therapy Catapult is an Innovate UK programme. The authors are employees of Cell and Gene Therapy Catapult. No writing assistance was utilized in the production of this manuscript.
References
1. ARM Q3 2016 data report. Website
2. U.S. Department of Health and Hu¬man Services, Food and Drug Admin¬istration (2004) Guidance for indus¬try: PAT– a framework for innovative pharmaceutical development, manu¬facturing and quality assurance. 2004. Website
3. Rathore A, Bhambure R and Ghare V. Process analytical technology (PAT) for biopharmaceutical products. Anal. Bioanal. Chem. 2010; 398(1), 137–54. CrossRef
4. Elliott T, Billingham S, Bi J, Zhang H. Quality by design for biopharma¬ceuticals: a historical review and guide for implementation. Pharm. Biopro-cess. 2013; 1(1), 105–122. CrossRef
5. NIH SEER cancer statistics review 1975-2011. Website
6. Onea A & Jazirehi AR. CD19 chime¬ric antigen receptor (CD19 CAR)-re¬directed adoptive T-cell immuno¬therapy for the treatment of relapsed or refractory B-cell Non-Hodgkin’s Lymphoma. Am. J. Cancer Res. 2016; 6(2), 403–24.
7. Rathore A, Bhambure R, Ghare V. Process analytical technology (PAT) for biopharmaceutical products. Anal. Bioanal. Chem. 2010; 398(1), 137–54.
CrossRef
8. Read E, Shah R, Riley B, Park J, Bror¬son K, Rathore A. Process analytical technology (PAT) for biopharmaceu¬tical products: Part II. Concepts and applications. Biotechnol. Bioeng. 2010; 105(2), 285–95. CrossRef
9. Mercier SM, Diepenbroek B, Wijffels RH, Streefland M. Multivariate PAT solutions for biopharmaceutical cul¬tivation: current progress and limita¬tions. Trends Biotechnol. 2014; 32(6), 329–36. CrossRef
10. Langfelder P, Horvath S. WGCNA: an R package for weighted correlation network analysis. BMC Bioinformatics 2008; 9, 559. CrossRef
11. Shukla A & Gottschalk U. Single-use disposable technologies for biophar¬maceutical manufacturing. Trends Biotechnol. 2013; 31(3): 147–54.
CrossRef
12. Rathore A, Mendhe R and Krull S. Tools for Enabling Process Ana¬lytical Technology Applications in Biotechnology. Biopharm. Int. 2012; 25(8).
13. Abu-Absi N, Kenty B, Cuellar M et al. Real Time Monitoring of Multiple Pa¬rameters in Mammalian Cell Culture Bioreactors Using an In-Line Raman Spectroscopy Probe. Biotech. Bioeng. 2011; 108(5), 1215–21. CrossRef
14. Weichert H, Lüders J, Becker M, Ad¬ams T, Weyand J. Integrated Optical Single-Use Sensors: Moving Toward a True Single-Use Factory for Biologics and Vaccine Production. Bioprocess Int. 2014; 12.
Affiliations
Damian Marshall1, Stephen Ward2 & Marc-Olivier Baradez1
1 Analytical Development, Cell and Gene Therapy Catapult, UK
2 Chief Operating Officer at Cell and Gene Therapy Catapult, UK
This work is licensed under a Creative Commons Attribution- NonCommercial – NoDerivatives 4.0 International License.